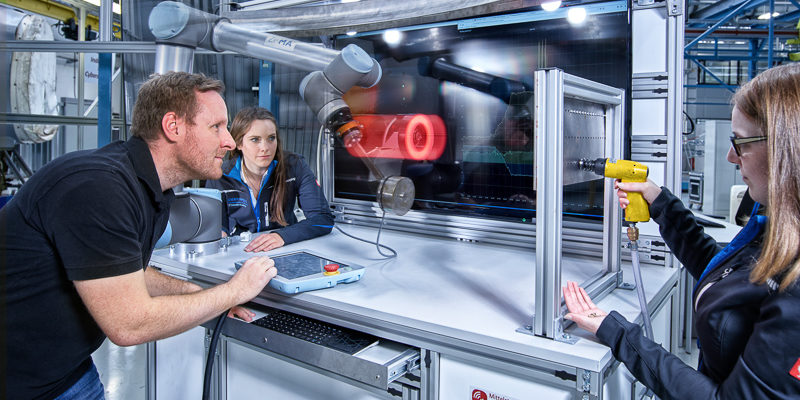
Problem definition
Quality assurance often takes place under poor ergonomic conditions. In addition, quality assurance is often manual, incomplete and analogue.
Advantages of the solution
Quality inspection can be performed during the process. Process parameters can thus be adjusted quickly and continuously.
Used technologies
Human-robot collaboration in process control, sensor data fusion of camera, laser line sensor and force-moment sensor as well as AI algorithms. Smart devices for visualisation.
Application areas
Quality assurance during the production process. The concept is transferable in many ways.
Aircraft production is facing various technical challenges, such as large product dimensions, complex joining processes and the organization of assembly tasks. Meeting the requirements that come with large dimensions, low tolerances and small batch sizes, in combination with complex joining processes, automation and labor-intensive inspection task, is often difficult to achieve in an economically viable way.
AQI-Inspect is an extension to the existing demonstrator “HRC Aircraft Riveting Process” (https://robot-hub.org/project/hrc-aircraft-riveting-process/). Existing sensors and actuators of the structure were used to reduce the workload and improve quality control in aircraft construction. Currently, quality control still consists largely of manual and monotonous tasks under poor ergonomic conditions. Only a bullet point and analogue quality control is carried out.
By equipping the tool with sensors, such as a force torque and laser line sensor, it can not only monitor the riveting process in real-time, but also perform inspection tasks using an artificial intelligence algorithm. The sensor data will be passed through a trained machine learning model classifying whether the scanned part is of good or bad quality. With a limited amount of data, the “online” rivet classification using sensor data reaches a classification precision of 86%, whereas the rivet classification based on pictures reaches a precision of 97%. The result of the quality inspection will be sent to the employee using Mixed Reality glasses. This will allow the operator to react appropriately and carry out necessary maintenance.
The concept can be applied to other areas as well. A key focus was to use existing resources in a new context to generate added value.
The results are part of the European Union’s Horizon 2020 research and innovation program at ZeMA